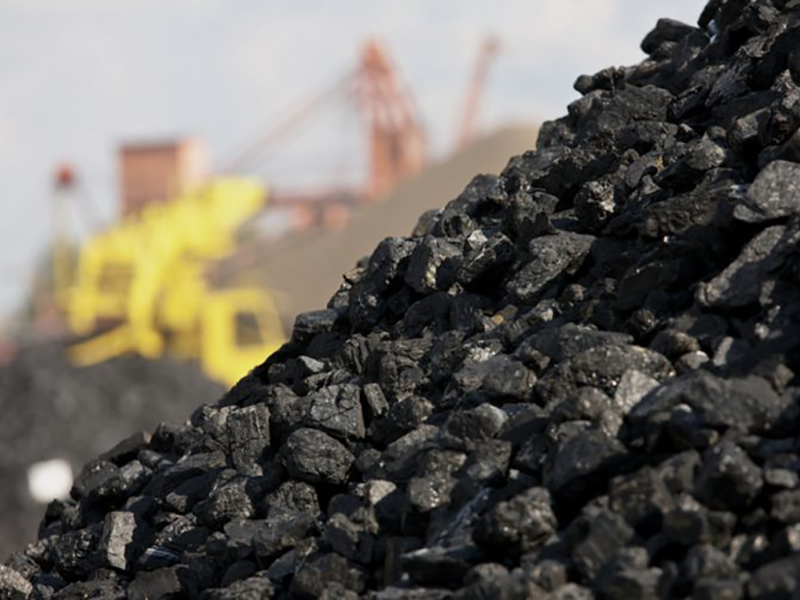
Oğuzhan Akyener, Raif Kaya, Efe Düveroğlu
Abstract
This study investigates whether there is a price linkage between energy resources such as crude oil, natural gas, and coal. Thus, the cointegration theory is applied to the Austrian and European monthly price series of Brent oil, natural gas, and coal in a relatively large sample of 40 years from 1980 to 2020. Based on the Time Series Analysis result, two cointegration approaches are used in this research: Engle-Granger and Philips Ouliaris Tests. Moreover, to examine the variability prices of crude oil, natural gas, and coal reflect a foreseeable casualty for each other, Granger Causality Test is also applied. To confirm the number of cointegration relationships, error correction mechanisms, and co-efficiency, Vector Auto Regression, Vector Error Connection, and Autoregressive Distributed Lag models are used. Even though cointegration theory expects that individual series can be non-stationary, a linear combination of those series should be stationary; this study’s results show no cointegration between energy prices in all cases.
INTRODUCTION
Energy is a vital condition of production in every aspect of the economic sector. Crude oil is incontestably one of the most significant energy commodities for the economic development and growth of industrialized and developing countries’ industries where many consumed petroleum products are refined. Over the years, the global demand for crude oil has remarkably increased due to the growing production demand of non-OECD countries, especially China, which is in a trade war with the United States (Hamilton, 2009). Natural gas and coal are mainly used in the electricity generation process. The balance of supply and demand has recently been undergoing a significant transformation in parallel with rising crude oil demand (Panella, 2012). As mentioned, even though crude oil, natural gas, and coal are the fundamental energy commodities for states, the supply of these commodities may not always meet the demand because energy commodity price dynamics are affected by several factors such as political conditions, state regulations, extreme weather conditions or even the competitive market behavior. Given that, the energy commodity prices have fluctuated catastrophically many times in history. At this point, the first question that comes to mind is, is the possible price change in one of the energy commodities a predictable scenario for the others?
Therefore, this study examines whether the prices of crude oil, natural gas, and coal are cointegrated. Cointegration theory allows researchers to analyze stationary non-stationary series by using a linear combination between the series. Thus, the cointegration analysis method enables the examination of both long-term stable relationships and short-term dynamics between specific series at the same time (Plaquet, 2015). To analyze the link between those three energy price series from 1980 to 2020, we use two main cointegration frameworks as, the Engle-Granger (1987) and Philips-Ouliaris (1990) methods.
This study is developed in five sections. In the second section that follows, a brief literature review is given, including several different studies on energy commodity prices. The third section describes the methodology of Engle-Granger and Philips-Ouliaris Cointegration Tests. The fourth section presents analysis and empirical results, and the last section reflects an overview of the results and conclusion.
LITERATURE REVIEW
The cointegration theory is based on fact that the several financial and macroeconomic features have a non-stationary nature. When common regression methods are applied to such series rather than cointegration methods, a problem called spurious regression is encountered due to misidentifying the variables that are statistically significant but unrelated (Granger & Newbold, 1974). While the application of cointegration methods the possibility of spurious regression, it also allows the examination of non-stationary series that is, in fact, stationary through applying linear combination (Engle & Granger, 1991; Meuriot, 2016). Thus, cointegration methods enable the analysis of the intensity of long-term equilibrium (stationary) and short-term factors (adjustment) between two or more series.
The cointegration methods have been used in several empirical analyzes of energy prices. In particular, analyses of cointegration relations between energy commodities such as crude oil, natural gas, and coal dominate the literature. For instance, Asche et al. (2001) analyzed the degree of market integration of natural gas imports in Belgium, France, and Germany between 1990 and 1997 by applying the Johansen test. The cointegration tests displayed that different border prices for natural gas to France have proportionally over time and without significant differences between stated countries. Therefore, the study concluded that national natural gas markets in countries were highly integrated.
Moreover, Bencivenga et al. (2010) examined the degree of crude oil, gas, and electricity prices in European markets. This research used the time series for the Brent crude oil, British natural gas, and the European Energy Exchange for electricity between 2001 and 2007, and the Engle-Granger method was used. Finally, the study indicated that European oil, gas, and electricity markets are integrated.
By adopting a more comprehensive approach, Plaquet (2015) investigated the cointegration among the European price series of crude oil, natural gas, and coal by engaging a relatively large sample from 1980 to 2015 and using Engle-Granger and Johansen models. Both models showed that even though there is a structural break in the models, there is a cointegration with a long-term relationship between energy commodity prices.
Apart from the studies mentioned and perhaps a few additional studies, very little empirical work has been done on the scope of the energy resources market in Europe. However, several methodological methods have been used in various studies on the American energy market. In this regard, Serletis and Herbert (1999) investigated whether Northern American oil, natural gas, and electricity markets are linked. In their study, scholars mainly focused on Henry Hub and Transco Zone 6, which includes four locations, and energy prices and used daily data from 1996 to 1997. Using the Engle-Granger methods, the study revealed a significant integration between oil and gas, but not for electricity.
On the same subject and in the same context, Bachmeier and Griffin (2006) analyzed the degree of market integration between crude oil, natural gas, and coal markets by using the daily price data from the five locations price in the United States. Following the results, oil prices in five locations are highly integrated into the economic market. However, coal prices are cointegrated, but the degree of market integration is fragile, especially between the Western and Eastern locations. Moreover, the results also showed that only crude oil and natural gas display cointegration in the long term.
Always on the same subject, Mohammadi (2009) examined the cointegration relationship between crude oil, natural gas, coal, and electricity prices in the United States by analyzing a large sample from 1960 to 2007. Unexpectedly, this study revealed that prices of energy commodities do not significantly affect the electricity prices in the United States, and a long-run relationship is only seen between electricity and coal prices. Furthermore, there seems to be no unified energy market in which electricity, coal, natural gas, and crude oil prices are affected.
Lastly, Mazoor and Seiflou (2011) investigated the cointegration between oil, gas, and coal prices using data from the American energy market between 1983 and 2008, involving 301 observations. Using an ARDL approach, the study found that even though there is a long-run relationship between oil, gas, and coal prices, gas prices do not have any effect on oil prices in the short-run.
METHODOLOGY
There are various causality and cointegration methods which investigate cointegration relationships among economic variables and energy indicators. The most common methods are Engle and Granger based on residuals, the Phillips and Hansen with a modified ordinary least square procedure, Johansen, and the Johansen and Juselius maximum likelihood methods (Mazoor and Seiflou, 2011).
The autoregressive distributed lag (ARDL) method or bounds test was initiated by Pesaran and Shin, (1999) and further developed by Pesaran et al., (2001). It is regarded as one of the most flexible methods in the econometric analysis of the energy-growth nexus, particularly when the research framework is shaped by shocks and regime shifts that change the pattern of energy consumption or the evolution of other involved variables in the models. Moreover, the fact that the ARDL method may accommodate different lags in different variables renders the method very attractive and underlines its flexibility. The accommodation of sufficient lags enables best capturing of the data generating process mechanism. More specifically the method can be applied irrespective of whether the time series is I(0), namely, stationary at levels, or I(1), namely, stationary at first differences or frictionally integrated (Menegaki, 2021).
The essence models in the ARDL bounds test framework are the following- unrestricted error correction models.
Δ is the first difference operator and μ is the error term that must be a white noise, or put in other words, it represents the residual term that is supposed to be well behaved (serially independent, homoskedastic and normally distributed).All α and β coefficients are nonzero with a4 and β4 also being negative (this represents the speed of adjustment). The parameters α2i and a3i represent the short-run dynamic coefficients, while a4 and a5 are long-run coefficients in the energy-growth nexus relationship. α0 and β0 are drift components, μ1t and μ2t are white noise. Overall, in the equation each variable is represented as dependent on the past values of itself, the past values of the other variable(s), and the past values of differenced values of itself and the past values of differenced values of the other variable(s).the equations can be formulated either as intercept or trend ARDL models or both.
μ1t and μ2t are the error terms with E(ut I Yt – 1, Yt – 2, ………., Xt – 1, Xt – 2, …….) = 0. The assumption that the errors in the ARDL model have a conditional mean of 0 given all past values of Y and X—that is, that E(ut I Yt – 1, Yt – 2, ………., Xt – 1, Xt – 2, …….) = 0implies that no additional lags of either Y or X belong in the ADL model (Stock and Watson, 2019).
TIME SERIES TESTS and ANALYSIS
Crude oil, coal and gas industries have different marketing ranges and properties. While crude oil has a wider and global marketing structure, usually coal and gas act more regionally and locally. This fact naturally effects the pricing mechanisms and tendencies. By considering this situation, it is important to select the most possibly related markets for evaluations.
In this regard, from the historical data availability and the market volumes,
- For crude oil markets, Brent is selected due to be utilized in a wider region and a higher share,
- For coal, as being one of the biggest and stable market, Australian coal is selected.
- For gas, due to being the biggest, much transparent and maturated market, European gas is selected.
Moreover, we have to note that, Brent referenced pricing is used in Australia and Europe regions. This also increases the possibility of these three different prices to have a higher level of relations.
In this regard, we have selected the Brent, Australian coal and Europe gas prices’ time series for analysis. In addition, we started with the basic price vs. time analysis.
As can be seen from the graph above, a slight relation and similar tendencies in the due prices of these different energy markets can be observed.
Moreover, in the graph below, the due energy prices were re-calculated according to their calorific values. And a shifting balance between these resources could be observed. By the way, the results of the latest energy crisis on gas prices could easily be understood.
After having general evaluations for the historical prices of these energy types, time series analysis was tried to be applied by using different test methods in E-Views Software.
Unit Root Test
Firstly, in order to be able to have a better look, we initially calculated the log values for each data. After that, we applied the unit root test for each time series.
In this regard, we selected the Augmented Dickey-Fuller Test method for evaluating the existence of unit root among due series and max lag has taken as 18.
Table 1: Crude Brent Unit Root Test Results (for log values)
Augmented Dickey-Fuller (ADF) test | t- Statistic | Prob. |
Crude Brent Unit Root Test Results | -1.88 | 0.34 |
Coal Unit Root Test Results | -0.49 | 0.89 |
Natural Gas Unit Root Test Results | -1.64 | 0.46 |
As can be seen from the tables above, all time series have observed as being non-stationary. Hence, P values for all cases were higher than 5 percent. So, null hypothesis was rejected. That is why; we understood that none of the series does not have unit roots.
Due to this fact, we continued by evaluating the first difference values for all series. As the result, we observed that at the first difference level, all series became stationary (while observing P values are lower than 5 percent, as can be seen in the table below).
Table 2: Oil, Coal and Natural Gas First Differences Unit Root Test Results (for log values)
Augmented Dickey-Fuller (ADF) test | t- Statistic | Prob. |
Crude Brent Unit Root Test Results | -17.27 | 0.00 |
Coal Unit Root Test Results | -16.83 | 0.00 |
Natural Gas Unit Root Test Results | -9.24 | 0.00 |
Engle Granger Cointegration Test
In previous analysis. by checking the unit root test results. we observed that the due studied crude oil. gas and coal prices are nonstationary. That is why. in this part we applied Engle Granger and Philips Ouliaris Cointegratiaon tests to understand if the combination of these series may act as stationary.
Firstly. Engle Granger Cointegration Test was applied to the group data of these price series.
Table 3: Engle Granger Cointegration Test Results
Dependent | tau-statistic | Prob. | z-statistic | Prob. |
LOGBRENT | -5.24 | 0.00 | -62.82 | 0.00 |
LOGCOAL | -3.03 | 0.23 | -21.73 | 0.11 |
LOGGAS | -5.32 | 0.00 | -61.70 | 0.00 |
While checking the P results given in Engle Granger Test. we can see that log coal’s probability value is greater than 5 percent. This means. Brent and gas prices are cointegrated. however coal does not fix within this cointegration.
Philips Ouliaris Cointegratiaon Test
After observing the results taken by Engle Granger Cointegration Test. secondly. Philips Ouliaris Cointegratiaon Test was applied to the group data of these price series.
Table 4: Philips Ouliaris Cointegratiaon Test Results
Dependent | tau-statistic | Prob. | z-statistic | Prob. |
LOGBRENT | -4.71 | 0.00 | -49.22 | 0.00 |
LOGCOAL | -2.74 | 0.36 | -17.64 | 0.22 |
LOGGAS | -4.88 | 0.00 | -50.55 | 0.00 |
While checking the P results given in Philips Ouliaris Test. we can see that log coal’s probability value again is greater than 5 percent. which means. again Brent and gas prices are cointegrated but. coal again does not fix within this cointegration.
Granger Causality Test
Engle Granger and Philips Ouliaris Cointegratiaon Tests were applied to the group data of these price sets. However. we observed that the combination of these series are non-stationary.
At this point. Granger Causality Test was applied for the same group of data and the causality results were given below.
Initially by accepting the lag value as 12 (hence the data is monthly). Granger Causality Test was applied to the log price group of coal. Brent and gas.
Table 5: Granger Causality Test Results
Obs | F-Statistic | Prob. | |
LOGCOAL does not Granger Cause LOGBRENT | 506 | 1.97 | 0.03 |
LOGBRENT does not Granger Cause LOGCOAL | 1.75 | 0.05 | |
LOGGAS does not Granger Cause LOGBRENT | 506 | 2.32 | 0.00 |
LOGBRENT does not Granger Cause LOGGAS | 6.56 | 6.E-11 | |
LOGGAS does not Granger Cause LOGCOAL | 506 | 2.56 | 0.00 |
LOGCOAL does not Granger Cause LOGGAS | 2.99 | 0.00 |
As can be seen in the results in the table above.
– We reject the null hypothesis: logcoal does not Granger Cause logbrent. Therefore. we understand that coal prices affect the brent prices. which also means; historical coal price data can be used in evaluation of current oil prices.
– We accept the null hypothesis: logbrent does not Granger Cause logcoal. Therefore. we understand that brent prices do not affect the coal prices. which also means; historical brent price data can not be used in evaluation of current coal prices.
– We reject the null hypothesis: loggas does not Granger Cause logbrent. Therefore. we understand that gas prices affect the brent prices. which also means; historical gas price data can be used in evaluation of current oil prices.
– We reject the null hypothesis: logbrent does not Granger Cause loggas. Therefore. we understand that brent prices affect the gas prices. which also means; historical brent price data can be used in evaluation of current gas prices.
– We reject the null hypothesis: loggas does not Granger Cause logcoal. Therefore. we understand that gas prices affect the coal prices. which also means; historical gas price data can be used in evaluation of current coal prices.
– We reject the null hypothesis: logcoal does not Granger Cause loggas. Therefore. we understand that coal prices affect the gas prices. which also means; historical coal price data can be used in evaluation of current gas prices.
Vector Auto Regression (VAR) Estimates
After the above analysis. we checked the VAR estimates. within the same price group.
After forming the lag structure. by using the lag length criteria was evaluated (by also taking the lag value as 12). Five different lag order selection criteria was observed. However. in this study. SC criteria was accepted as the reference case. So. the lowest SC value was observed in lag number 2. That is why. this VAR integrated new lag number was applied again in the Granger Causality Test.
New Granger Causality Test with Lag Number 2
The above Granger Causality Test was re-applied with lag number is equal to two.
Table 6: New Granger Causality Test Results (lag number is two.)
Obs | F-Statistic | Prob. | |
LOGCOAL does not Granger Cause LOGBRENT | 506 | 3.16 | 0.04 |
LOGBRENT does not Granger Cause LOGCOAL | 3.93 | 0.02 | |
LOGGAS does not Granger Cause LOGBRENT | 506 | 3.55 | 0.03 |
LOGBRENT does not Granger Cause LOGGAS | 16.31 | 1.E-07 | |
LOGGAS does not Granger Cause LOGCOAL | 506 | 2.61 | 0.07 |
LOGCOAL does not Granger Cause LOGGAS | 5.57 | 0.00 |
In addition. with these new results; as can be seen in the table above.
– We again reject the null hypothesis: logcoal does not Granger Cause logbrent. Therefore. we understand that coal prices affect the brent prices. which also means; historical coal price data can be used in evaluation of current oil prices.
– We now reject the null hypothesis: logbrent does not Granger Cause logcoal. Therefore. we understand that Brent prices affect the coal prices. which also means; historical Brent price data can be used in evaluation of current coal prices.
– We again reject the null hypothesis: loggas does not Granger Cause logbrent. Therefore. we understand that gas prices affect the Brent prices. which also means; historical gas price data can be used in evaluation of current oil prices.
– We again reject the null hypothesis: logbrent does not Granger Cause loggas. Therefore. we understand that Brent prices affect the gas prices. which also means; historical Brent price data can be used in evaluation of current gas prices.
– We now accept the null hypothesis: loggas does not Granger Cause logcoal. Therefore. we understand that gas prices do not affect the coal prices. which also means; historical gas price data can not be used in evaluation of current coal prices.
– We again reject the null hypothesis: logcoal does not Granger Cause loggas. Therefore. we understand that coal prices affect the gas prices. which also means; historical coal price data can be used in evaluation of current gas prices.
As a result. lag difference changed the results in our analysis.
VECM Test
Initially as can be shown in the table below. Vector Error Connection Model Estimates was analyzed by assuming initially the series are cointegrated. Lag number is taken as 2 due to the results of the previous studies.
Table 7: Vector Error Connection Model Results
Cointegrating Eq: | CointEq1 | ||
LOGBRENT(-1) | 1,00 | ||
LOGCOAL(-1) | 0,99 | ||
LOGGAS(-1) | -1,23 | ||
C | -2,09 | ||
Error Correction: | D(LOGBRENT) | D(LOGCOAL) | D(LOGGAS) |
CointEq1 | -0.031 | 0.07 | 0.05 |
(0.01) | (0.03) | (0.03) | |
[-2.27] | [ 2.26] | [ 1.40] |
As can be seen in the table above. the green values are the coefficient estimates for due series and the blue ones are the standard errors. Lastly. the yellow ones are the t test statistics. where; -for Brent and gas series. values are greater than |1.96|. which means they are significant. -but for coal. it is not significant.
ARDL Test
After analyzing the VECM estimates. ARDL test applied to the due series. Lag interval is taken as 12. due to the data is monthly. In addition our dependent variable is selected as the Brent. hence it is being the most influential market affecting the other prices. while comparing with the gas and coal.
Table 8: ARDL test Results (Dependent Variable: LOGBRENT)
Variable | t-Statistic | Prob.* |
LOGBRENT(-1) | 28,34 | 0,00 |
LOGBRENT(-2) | -6,51 | 0,00 |
LOGCOAL | 4,56 | 0,00 |
LOGCOAL(-1) | -3,99 | 0,00 |
LOGGAS | 0,45 | 0,65 |
LOGGAS(-1) | 0,54 | 0,59 |
LOGGAS(-2) | 0,56 | 0,58 |
LOGGAS(-3) | -1,90 | 0,06 |
LOGGAS(-4) | 0,57 | 0,57 |
LOGGAS(-5) | -0,53 | 0,60 |
LOGGAS(-6) | 0,25 | 0,81 |
LOGGAS(-7) | 1,43 | 0,15 |
LOGGAS(-8) | 0,36 | 0,72 |
LOGGAS(-9) | -0,72 | 0,47 |
LOGGAS(-10) | 0,09 | 0,93 |
LOGGAS(-11) | -2,91 | 0,00 |
LOGGAS(-12) | 3,96 | 0,00 |
C | 1,24 | 0,22 |
From the ARDL Bonds Test. we observed that d=1. which means in I(1) process can be activated and unit root exists.
Then. from view; we checked the coefficient diagnostics;
Table 9: Coefficient Diagnostics
Variable | Coefficient | Std. Error | t-Statistic | Prob. |
LOGCOAL | 0,44 | 1,85 | 2,35 | 0,02 |
LOGGAS | 0,78 | 0,17 | 4,55 | 0,00 |
C | 0,70 | 0,53 | 1,32 | 0,19 |
F-statistic | 4,99 | 10% | 2,63 | 3,35 |
2,00 | 5% | 3,10 | 3,87 | |
2.5% | 3,55 | 4,38 | ||
1% | 4,13 | 5,00 |
Where. F-statistic value (yellow) is greater than in all significance values (purple). then we conclude that; we can reject the null value. which means there is cointegration in all cases. except the %1 values.
RESULTS and DISCUSSION
As can be seen from the due time series analysis we can conclude that:
- Base level for all due time series are non-stationary. so; none of the series does not have unit roots.
- At the first difference level. all series became stationary.
- Engle Granger and Philips Ouliaris Cointegratiaon Tests show that the combination of these series are non-stationary.
- According to “New Granger Causality Test with Lag Number 2” results:
- Coal prices affect the Brent prices.
- Brent prices affect the coal prices.
- Gas prices affect the Brent prices.
- Brent prices affect the gas prices.
- Gas prices do not affect the coal prices.
- Coal prices affect the gas prices.
- According to VECM test.
- For Brent and gas series. t-test statistics are significant.
- for coal. it is not significant.
- According to ARDL method. there is a general cointegration among the due energy prices.
Actually. as mentioned and discussed in the introductory part. these results can be expected by overviewing the dynamics of the due sectors. Tendencies in oil. gas and coal markets are different. Moreover. although the world has been on a trend of gasification instead of coal utilization in electricity production for more than 20 years. it is not easy to say these resources are completely substitutes between each other. Moreover. oil has a more widely integrated marketing structure by comparing the others. However. climate change concerns. current supply crisis. increase in the trade of LNG form and calorific value indexed hub energy mechanisms step by step make these energy types become a more cointegrated position.
As a result. as we have observed different results with different tests above. by interpreting for a long time equilibrium. we can say that;
- These three different types of prices are not completely cointegrated.
- oil and gas prices shows a better interrelation between each other. by taking the coal out of the analysis.
- Crude oil prices have a higher effect on the gas prices.
- in crisis times. a more complex interrelation among these three resources can be observed for a shorter time period.
CONCLUSION
Oil, gas and coal are the three important black energy resources influencing more than 75 percent of global energy demand. As can naturally be observed in the crisis times an increase in one of these prices result in an upward effect on the other prices. That is why; it becomes important to understand the possible relation between these three different types of energy resources.
That is why, in this study we investigated whether there is a price linkage between energy oil gas and coal markets. In this regard around a 40 years time period was evaluated to interpret the possible long term relations. Based on the Time Series Analysis result two cointegration approaches are used in this research: Engle-Granger and Philips Ouliaris Tests. Moreover, to examine the variability prices of crude oil natural gas and coal reflect a foreseeable casualty for each other. Granger Causality Test is also applied. To confirm the number of cointegration relationships error correction mechanisms and co-efficiency. Vector Auto Regression. Vector Error Connection and Autoregressive Distributed Lag models are used. Even though cointegration theory expects that individual series can be non-stationary, a linear combination of those series should be stationary; this study’s results show not a whole cointegration between these combined energy prices. However, we could observe that oil and gas prices shows a better interrelation between each other. by taking the coal out of the analysis.
These results can be accepted from a practical evaluation of the sectors hence we can easily say that:
- Crude oil prices have higher influencing abilities on gas and slightly coal prices.
- In a long-term global overview, although somehow follow similar trends (according to economic conditions) these three markets act within different tendencies.
- However, this situation changes locally. For example in USA gas prices can be accepted as the 1/10 of the WTI prices from a general sight. By the way, this situation also can change in crisis times. This also means that from a local point of view oil prices can determine the gas prices. However, this case will not fit with the European markets’ tendencies.
- Due to these facts. it is not easy to say for a long time globally oil prices effects the gas prices. However, again we can accept that they have a general cointegration.
- By including the coal prices into the evaluations, we can observe that coal has a more separated pricing mechanism and breaks the existing cointegration between oil and gas.
- Coal can slightly be effected by oil and gas prices in crisis time but this situation cannot be accepted in a long-term global scheme.
REFERENCES
Hamilton. James D. “Understanding Crude Oil Prices.” The Energy Journal. vol. 30. no. 2. 2009. https://doi.org/10.5547/issn0195-6574-ej-vol30-no2-9.
Panella. Massimo. et al. “Forecasting Energy Commodity Prices Using Neural Networks.” Advances in Decision Sciences. vol. 2012. 2012. pp. 1–26.. https://doi.org/10.1155/2012/289810.
Plaquet. Alexandre. “Cointegration Analysis among European Crude Oil. Natural Gas and Coal Prices between 1980 and 2015.” Louvain School of Management. 2015.
Engle. Robert F.. and C. W. Granger. “Co-Integration and Error Correction: Representation. Estimation. and Testing.” Econometrica. vol. 55. no. 2. 1987. p. 251.. https://doi.org/10.2307/1913236.
Phillips. P. C.. and S. Ouliaris. “Asymptotic Properties of Residual Based Tests for Cointegration.” Econometrica. vol. 58. no. 1. 1990. p. 165.. https://doi.org/10.2307/2938339.
Granger. Clive W.J.. and P Newbold. “Spurious Regressions in Econometrics.” A Companion to Theoretical Econometrics. 1974. pp. 557–561.. https://doi.org/10.1002/9780470996249.ch27.
Meuriot. Véronique. “The Concept of Cointegration: The Decisive Meeting between Hendry and Granger (1975).” Cahiers D’économie Politique. n° 68. no. 1. 2016. pp. 91–118.. https://doi.org/10.3917/cep.068.0091.
Engle. R. F.. and J C W Granger. Long-Run Economic Relationships: Readings in Cointegration. Oxford University Press. 1991.
Bencivenga. Cristina. et al. “Energy Markets: Crucial Relationship between Prices.” Mathematical and Statistical Methods for Actuarial Sciences and Finance. 2010. pp. 23–32.. https://doi.org/10.1007/978-88-470-1481-7_3.
Asche. Frank. et al. “Market Integration for Natural Gas in Europe.” International Journal of Global Energy Issues. vol. 16. no. 4. 2001. p. 300.. https://doi.org/10.1504/ijgei.2001.000925.
Serletis. Apostolos. and John Herbert. “The Message in North American Energy Prices.” Energy Economics. vol. 21. no. 5. 1999. pp. 471–483.. https://doi.org/10.1016/s0140-9883(99)00015-8.
Bachmeier. Lance J.. and James M. Griffin. “Testing for Market Integration: Crude Oil. Coal. and Natural Gas.” The Energy Journal. vol. 27. no. 2. 2006. https://doi.org/10.5547/issn0195-6574-ej-vol27-no2-4.
Mohammadi. Hassan. “Electricity Prices and Fuel Costs: Long-Run Relations and Short-Run Dynamics.” Energy Economics. vol. 31. no. 3. 2009. pp. 503–509.. https://doi.org/10.1016/j.eneco.2009.02.001.
Manzoor. Davood. and Sajjad Seiflou. “Are Crude Oil. Gas and Coal Prices Cointegrated?” Iranian Economic Review. vol. 15. no. 28. 2011.
Stock, J. H., & Watson, M. W. (2019). Introduction to Econometrics. In Biometrics.
Menegaki, A. (2021). An A–Z guide for complete research when using the autoregressive distributed lag (ARDL) bounds test approach in the broader energy-growth nexus. In A Guide to Econometrics Methods for the Energy-Growth Nexus (Issue 2). https://doi.org/10.1016/b978-0-12-819039-5.00007-0